Table of Contents
Are you experiencing performance bottlenecks in your Python applications? Have you wondered why your multi-threaded Python code doesn't fully utilize all CPU cores? The answer lies in Python's Global Interpreter Lock (GIL). For years, this mechanism has been a significant limitation for CPU-bound tasks in Python, but Python 3.13 introduces a revolutionary change. In this blog post, we'll explore what the GIL is, how it impacts your Python applications, and the exciting new ability to disable it.
What is the Global Interpreter Lock (GIL)?
The Global Interpreter Lock (GIL) is a mutex in CPython (Python's most common implementation) that restricts multiple native threads from executing Python bytecode simultaneously. In simpler terms, the GIL ensures only one thread can execute Python code at any given time, regardless of how many CPU cores your system has.
When we run Python code, the interpreter translates it into instructions for execution. Every thread needs access to the interpreter to execute these instructions. The GIL acts as a gatekeeper, allowing only one thread at a time to use the interpreter. This means that even in multi-threaded programs, Python threads take turns executing rather than running simultaneously - this is the fundamental limitation imposed by the GIL.
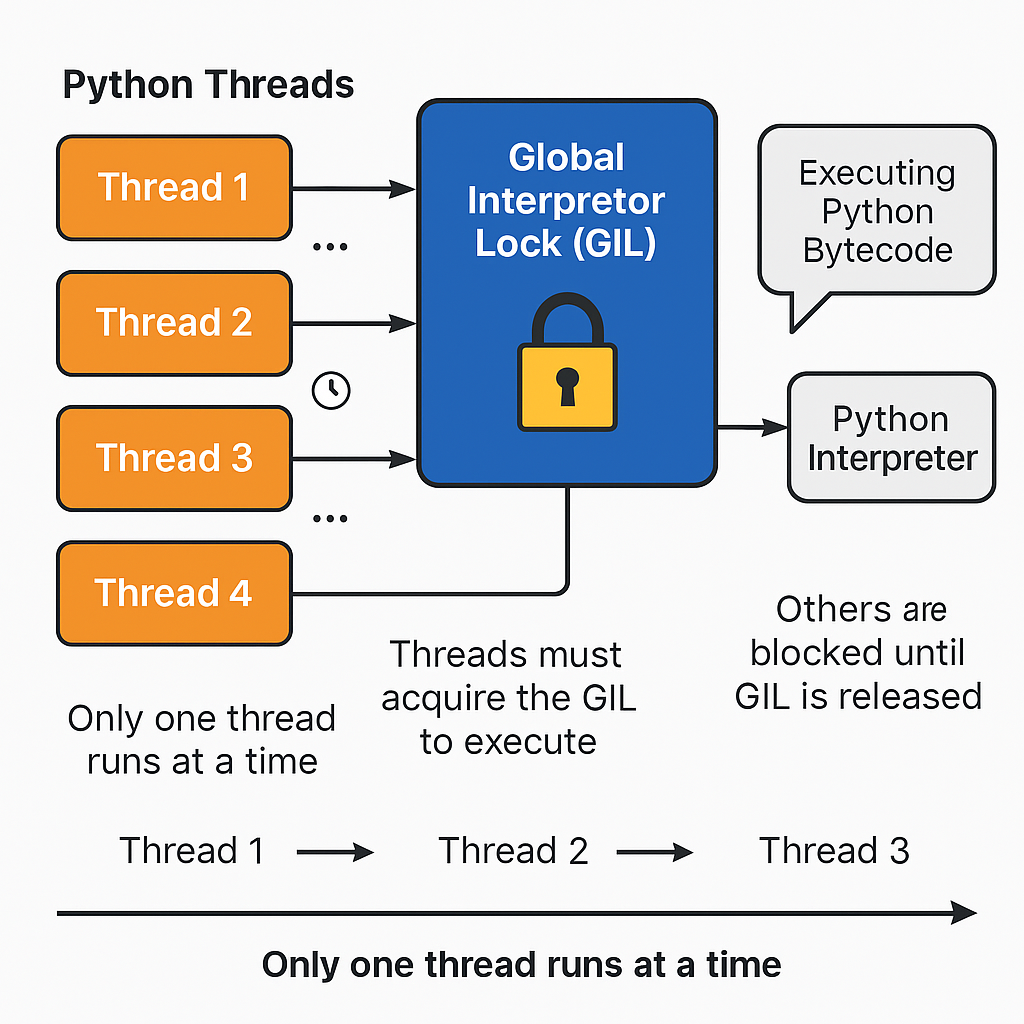
Why Does Python Use a GIL?
The GIL was introduced to solve specific problems:
- Memory Management Simplicity: Python uses reference counting for memory management. The GIL prevents race conditions when multiple threads might modify reference counts simultaneously.
- C Extension Compatibility: Many Python libraries utilize C extensions, and the GIL simplifies their integration by avoiding complex thread-safety mechanisms.
- Single-Threaded Performance: By avoiding the overhead of fine-grained locking, single-threaded Python programs run faster.
Existing Parallelism in Python Despite the GIL
While the GIL limits true thread-based parallelism, Python offers several approaches to achieve concurrent execution:
1. Threading
Python's threading
module supports multi-threading, but its effectiveness is limited by the GIL for CPU-bound tasks. However, threads still provide benefits for I/O-bound operations (like network requests or file operations) because the GIL is released during I/O waits.
import threading
def cpu_bound_task():
# This will be limited by GIL
result = 0
for i in range(10000000):
result += i
return result
# Creating multiple threads
threads = [threading.Thread(target=cpu_bound_task) for _ in range(4)]
for thread in threads:
thread.start()
for thread in threads:
thread.join()
2. Multiprocessing
The multiprocessing
module creates separate Python processes, each with its own interpreter and GIL, enabling true parallel execution. This approach is effective but comes with higher memory overhead and data serialization costs.
import multiprocessing
def cpu_bound_task():
# This can run in parallel
result = 0
for i in range(10000000):
result += i
return result
# Creating multiple processes
processes = [multiprocessing.Process(target=cpu_bound_task) for _ in range(4)]
for process in processes:
process.start()
for process in processes:
process.join()
3. C Extension Parallelism
Many high-performance Python libraries (like NumPy, Pandas, and SciPy) implement CPU-intensive operations in C code, which can release the GIL during execution, allowing for parallelism even in a GIL-constrained environment.
Disabling the GIL in Python 3.13
Python 3.13 introduces the option to disable the GIL through a special build called "Free-threaded CPython." This build allows Python threads to execute truly in parallel, potentially offering significant performance gains for multi-threaded, CPU-bound tasks.
How to Use Python Without the GIL
There are two main approaches to using GIL-free Python:
1. Installing Free-threaded CPython
You can specifically install the free-threaded Python build, which is separate from standard CPython. During installation, select the "Download free-threaded binaries" option to install Python 3.13t, where the GIL is already disabled.
Running programs with standard vs. free-threaded builds:
# Standard CPython build
python main.py
# Free-threaded CPython build
python3.13t main.py
2. Using Docker with Free-threaded Python
Another approach is to create a Docker container with free-threaded Python. This requires modifying the Dockerfile to include the --disable-gil
flag during configuration:
FROM buildpack-deps:bookworm
# Configure with GIL disabled
RUN ./configure \
--build="$gnuArch" \
--enable-loadable-sqlite-extensions \
--enable-optimizations \
--enable-option-checking=fatal \
--enable-shared \
--with-lto \
--with-ensurepip \
--disable-gil \
;
Checking GIL Status
You can verify whether the GIL is enabled or disabled in your Python environment with a simple script:
import sys
import sysconfig
def main():
# Check Python version
print(f"Python version: {sys.version.split()[0]}")
# Check GIL Status
status = sysconfig.get_config_var("Py_GIL_DISABLED")
if status is None:
print("GIL disabling is not supported in this Python version.")
elif status == 0:
print("GIL is active")
else:
print("GIL is disabled")
if __name__ == "__main__":
main()
Performance Benefits of Disabling the GIL
Removing the GIL can lead to substantial performance improvements for CPU-bound, multi-threaded tasks. Here's a simple example: calculating the Fibonacci number multiple times to get an average runtime on both single and multi-threaded workloads.
With GIL Enabled:
.venv-3.13.0krupakar@airflow-1:~/Blogs/python_GIL$ python main.py
Calculated Fibonacci(30) 20 times
Single-threaded execution time: 4.18 seconds
Calculated Fibonacci(30) 20 times
Multi-threaded (4 threads) execution time: 4.98 seconds
With GIL Disabled:
.venv-3.13.0tkrupakar@airflow-1:~/Blogs/python_GIL$ python main.py
Calculated Fibonacci(30) 20 times
Single-threaded execution time: 8.49 seconds
Calculated Fibonacci(30) 20 times
Multi-threaded (4 threads) execution time: 2.66 seconds
In this example, the GIL-disabled version executes the multi-threaded task nearly twice as fast as the GIL-enabled version. This performance gap widens with more cores and more computationally intensive tasks.
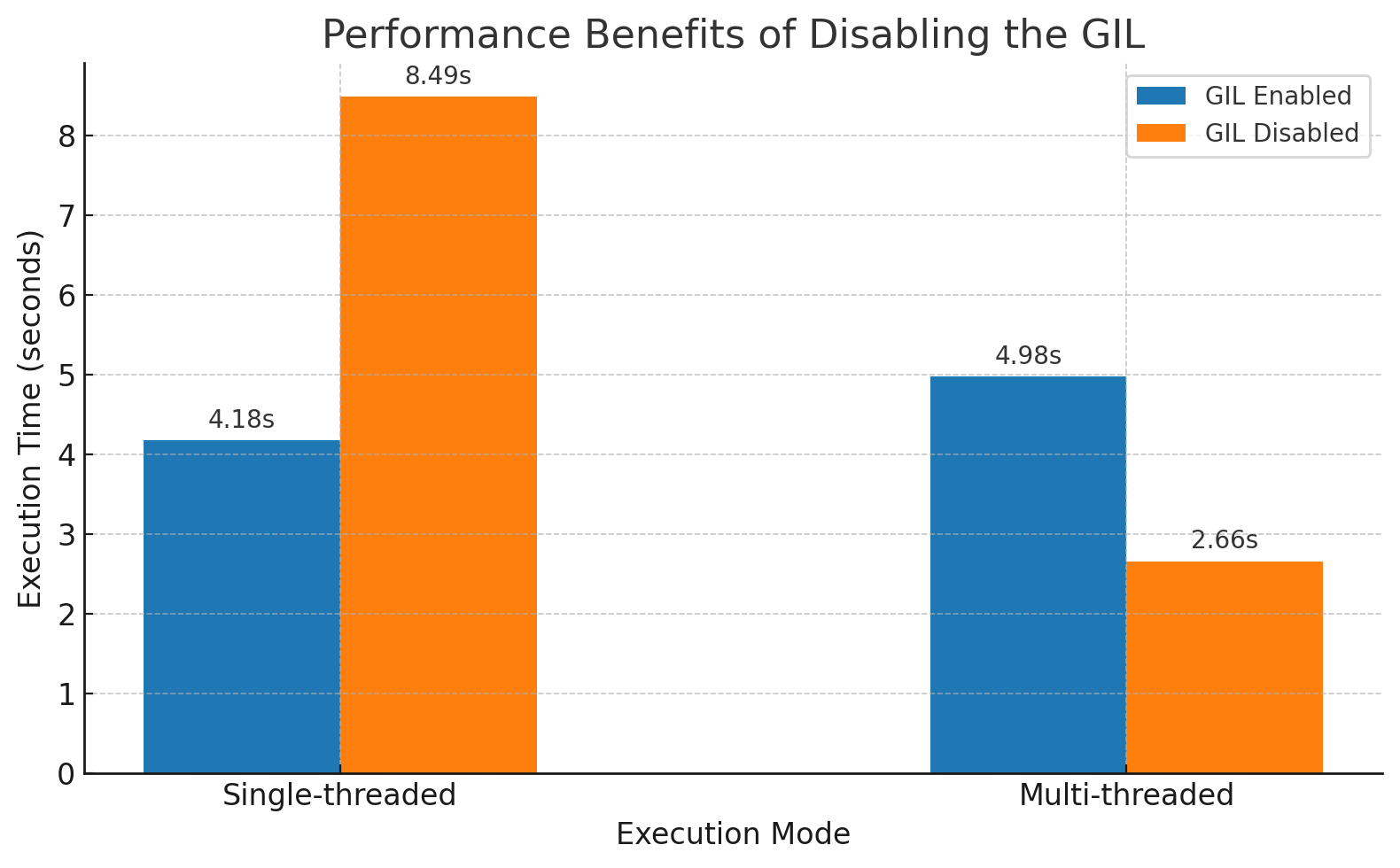
Problems with Disabling the GIL
While disabling the GIL unlocks true parallel execution, it also introduces a set of challenges and trade-offs that developers must consider before adopting it.
- Runtime Stability Risks
Removing the GIL fundamentally alters how Python manages memory and concurrency. This can destabilize the Python runtime and introduce hard-to-debug issues. Many parts of CPython's internals and ecosystem have been designed with the GIL in mind, and their assumptions may no longer hold true in a GIL-free environment. - Compatibility Challenges
Supporting both GIL-enabled and GIL-free Python builds increases complexity for package maintainers. Some extensions and modules—especially those interfacing with non-Python code—rely heavily on the GIL to provide implicit thread safety. These modules may behave unpredictably or even break when the GIL is removed. - Stop-the-World Pauses
To maintain consistency of internal data structures or during garbage collection, GIL-free Python can trigger stop-the-world events. These events pause all but one thread to ensure a consistent state. While necessary, they can negatively affect latency and responsiveness, especially in real-time or low-latency applications. - Performance Overhead for Single-Threaded Workloads
Without the GIL, additional synchronization mechanisms are needed to ensure thread safety. This overhead can degrade performance for single-threaded applications that previously benefited from the simplicity and speed the GIL offered. - Module Dependency on GIL Internals
Many Python libraries, particularly those with C extensions, assume the presence of the GIL for correctness and performance. These modules may exhibit bugs, crashes, or performance regressions when run under a GIL-free interpreter unless explicitly adapted for the new threading model.
Real-World Applications and Considerations
Disabling the GIL opens up exciting possibilities for Python in CPU-intensive domains:
- Machine Learning and Data Science: Faster data preprocessing and model training when using custom algorithms.
- Scientific Computing: More efficient simulations and numerical computations across multiple cores.
- API Servers: Improved throughput for compute-heavy API endpoints.
- ETL Workflows: Faster data transformation processes when dealing with large datasets.
However, there are important considerations:
- Thread Safety: Without the GIL, developers must explicitly handle thread safety using locks and other synchronization mechanisms.
- Library Compatibility: Not all libraries are tested or optimized for GIL-free operation yet. Some may automatically re-enable the GIL or experience issues.
- Single-Threaded Performance: GIL-free Python might perform slightly slower for single-threaded applications due to the added overhead of thread-safety mechanisms.
The Future of GIL-Free Python
The option to disable the GIL in Python 3.13 is still experimental and not recommended for production use yet. Many widely used packages like Pandas, Django, and FastAPI have not been fully tested in a GIL-free environment and may exhibit stability or performance issues.
Based on current development trajectories, we might expect:
- GIL-free Python to become stable around Python 3.16 or 3.17
- Wider library support for GIL-free operation in the coming years
- Potential for GIL-free mode to become the default in Python 3.20 or later
Until then, it's an exciting development primarily for testing and specific use cases where the performance benefits clearly outweigh the risks.
Conclusion
The ability to disable the Global Interpreter Lock in Python 3.13 represents a significant milestone in Python's evolution. This feature finally addresses one of Python's most persistent limitations by unlocking true multi-threading capability, potentially revolutionizing performance for CPU-bound tasks. The benchmarks clearly demonstrate the substantial performance gains possible when threads can execute in parallel.
In real-world applications, this change makes Python an even more viable option for computationally intensive workloads that previously might have required different languages or complex workarounds. Organizations leveraging data science, machine learning, or high-performance computing can now consider Python solutions that fully utilize their multi-core infrastructure without sacrificing the language's readability and extensive ecosystem.
If you're considering how to optimize your Python applications for multi-core performance or evaluating whether GIL-free Python could benefit your specific use cases, KubeNine Consulting can help. Our team of DevOps and cloud experts specializes in system architecture, infrastructure optimization, and performance tuning across diverse cloud environments. Contact us today to discover how we can help you implement cutting-edge Python solutions that maximize efficiency and scalability in your specific domain.