Table of Contents
Introduction
AI has transformed from a buzzword into an essential toolkit. AI capabilities continue to expand at a mind-boggling pace.
While AI Agents are great, do you know what's even better? AI Workforces. What if you could simply create multiple agents with different roles and responsibilities? These agents could work together autonomously to solve complex problems using tools provided them to them - they could critique each other, collaborate, and even delegate tasks to each other.
This is not a nobel concept - a lot of folks are already doing this, but we need a simple framework to make it easier for everyone. While doing our research we stumbled across CAMEL-AI and we were blown away by the potential.
What Is CAMEL-AI
CAMEL-AI (Communicative Agents for Machine Learning) is a framework that cuts through the complexity of creating collaborative AI systems. It's essentially a simple Python library that lets you orchestrate intelligent conversations between multiple AI agents with minimal setup time.
What I love about CAMEL is its stateful memory system - agents can actually remember context from previous interactions, making their decision-making much more coherent over extended conversations. For someone who values automation and efficiency (like I do with infrastructure), CAMEL brings that same philosophy to agent-based AI development.
Why Camel AI?
What truly sets CAMEL apart is its workforce capabilities. Just as I've built teams of specialists to handle complex infrastructure projects, CAMEL lets you create coordinated teams of AI agents with specialized roles and responsibilities.
You can define hierarchies, communication channels, and task delegation patterns.
For example, I recently experimented using Camel to create an SEO agency using CAMEL's workforce features. I defined agents with different areas of expertise - one focused on technical SEO, another on content analysis, and a third on UX. The agency chair agent coordinated their efforts and synthesized a final report that was remarkably thorough and effective.
Creating Our First Agent
Lets begin working with Camel AI with a simple example to get started. Open up a code editor of your choice and make sure python is installed. Here’s how the workflow will look.
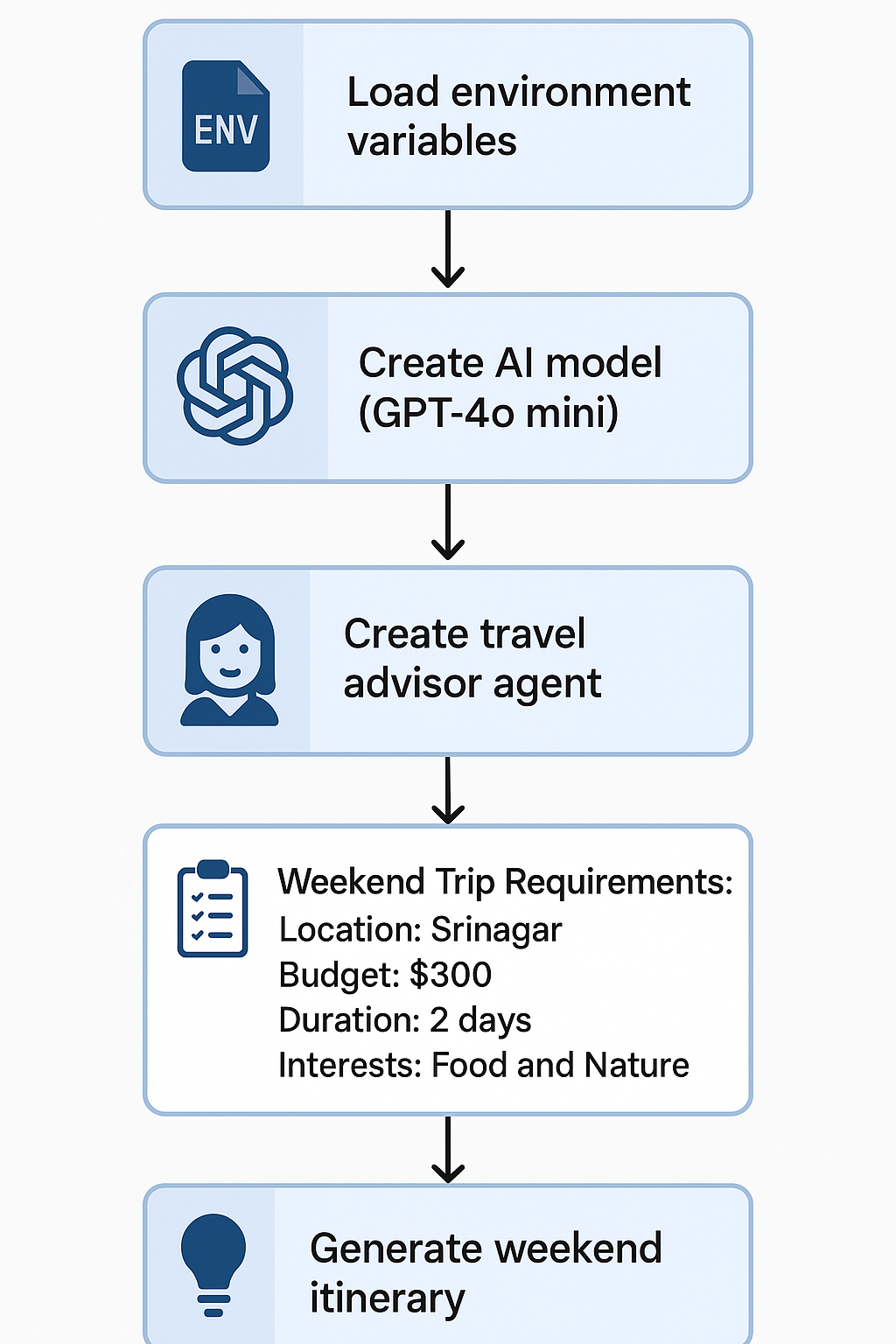
Install Dependencies
- Create and activate a virtual environment by running the command below in your terminal.
python -m venv venv
source venv/bin/activate #for ubuntu
venv/Scripts/activate #for windows
source .venv/bin/activate #for MacOS
- Install Camel AI library
pip install camel-ai[all]
Setup API Keys
- Create a .env file to stole environment variables, OpenAI API key in this case.
OPENAI_API_KEY = sk-proj-....
Import Environment Variables
- Create a main.py file, this file will hold all the logic, import the API key here
import os
load_dotenv()
secret_key = os.getenv("OPENAI_API_KEY")
Define the model
- Here we define a model that we want to use for our agents. Camel AI supports a wide range of agents like Deepseek, Llama models, GPT Models and many more. In this example we use GPT-4o mini as the model
from camel.models import ModelFactory
from camel.types import ModelPlatformType, ModelType
from camel.configs import ChatGPTConfig
model = ModelFactory.create(
model_platform=ModelPlatformType.OPENAI,
model_type=ModelType.GPT_4O_MINI,
model_config_dict=ChatGPTConfig().as_dict(),
)
Set Chat Agent
Lets say we want to create an agent which works as a travel advisor, you can define the agent as shown below
from camel.agents import ChatAgent
travel_advisor = ChatAgent(
system_message="""You are a friendly travel advisor who helps people plan weekend trips.
You give short, practical advice and always consider the budget.""",
model=model
)
‘system_message’ is the prompt that the agent will follow to work on the task, it will follow the ‘model’ to carry out these tasks.
Give Evaluation Criteria
In this case we’ll give trip details as the evaluation criteria. This will act as the set of rules the agent will evaluate your requests on.
trip_details = """
Weekend Trip Requirements:
- Location: Srinagar
- Budget: $300
- Duration: 2 days
- Interests: Food and Nature
"""
Interact with the Agent
print("Travel Advisor's Suggestions:")
response = travel_advisor.step(f"Please suggest a weekend itinerary based on: {trip_details}")
print(response.msgs[0].content)
This is the final step, the agent will now look into evaluation criteria and give the response accordingly.
The output can be seen here:
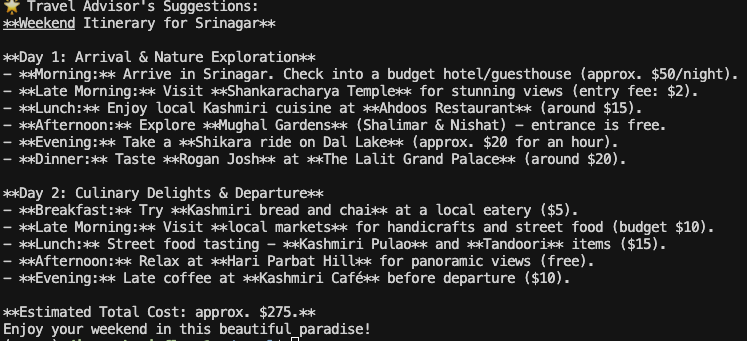
This was just a small glimpse of the abilities of Camel AI, if used correctly, it can handle much larger and more complex tasks with ease.
Camel AI for Real Life Use-case
We at KubeNine had a comprehensive task to evaluate a particular website from start to end, we had to find out how the website performed under various conditions, evaluate its UI UX, check for SEO optimizations and many more things.
Trying to do this manually would have cost us a lot of time and resources, so we decided to make use of Camel AI to help us make it easier for us, and it helped us a lot.
Roadmap
Since we had to evaluate different criteria, we made different agents each with their own specialities to help us with it. This is how we did it:
Setting Up the environment
import os
from dotenv import load_dotenv
import textwrap
from camel.agents import ChatAgent
from camel.messages import BaseMessage
from camel.models import ModelFactory
from camel.tasks import Task
from camel.toolkits import FunctionTool, SearchToolkit
from camel.types import ModelPlatformType, ModelType
from camel.societies.workforce import Workforce
import requests
from bs4 import BeautifulSoup
from urllib.parse import urljoin
Setting Up Agents
We set up multiple agents with different personas for evaluating the website. But first, we create a skeleton for all the agents to work with, we will call it ‘judge’. Think of this as "personality creator" for AI agents. It's like creating different characters for different roles.
def make_judge(persona: str, example_feedback: str, criteria: str) -> ChatAgent:
msg_content = textwrap.dedent(
f"""\
You are a judge evaluating a website.
This is your persona that you MUST act with: {persona}
Here is an example feedback that you might give with your persona:
{example_feedback}
When evaluating websites, you must use the following criteria:
{criteria}
You also need to give scores based on these criteria, from 1-4. The score given should be like 3/4, 2/4, etc.
"""
)
sys_msg = BaseMessage.make_assistant_message(
role_name="Website Evaluator",
content=msg_content,
)
model = ModelFactory.create(
model_platform=ModelPlatformType.OPENAI,
model_type=ModelType.GPT_4O_MINI,
)
return ChatAgent(system_message=sys_msg, model=model)
Each agent will now have 3 main parts:
- Persona: Who they are (like their job title and personality)
- Example feedback: How they should give feedback
- Criteria: What they should look for
- SEO Agent
seo_agent = make_judge(
persona="SEO Expert Sarah, a meticulous SEO specialist with 10 years of experience",
example_feedback="The meta descriptions are poorly optimized, and the keyword density needs work. I noticed several missed opportunities for internal linking.",
criteria="""
1. Meta tags optimization
2. Keyword usage and placement
3. URL structure
4. Internal linking
5. Content optimization for search
"""
)
Now that we have the judge ready, we can pass different parameter to it to personalize it as per our needs. To create an SEO evaluation agent, we gave it a persona which it will inherit, an example feedback so that it knows how to evaluate the content and gave it the criterion it should work with. Now that we know how to do this, we can create the other agents similarly.
- Content Agent
content_agent = make_judge(
persona="Content Creator Chris, a content strategist who focuses on engagement and clarity",
example_feedback="The content lacks a clear hierarchy and the messaging isn't consistent across pages. The value proposition needs to be more prominent.",
criteria="""
1. Content clarity and readability
2. Message consistency
3. Value proposition
4. Content structure
5. Engagement factors
"""
)
- Performance Agent
performance_agent = make_judge(
persona="Performance Pete, a technical expert obsessed with website speed and optimization",
example_feedback="The image sizes aren't optimized, and there's no proper caching implementation. The page load time could be significantly improved.",
criteria="""
1. Page load speed
2. Resource optimization
3. Code efficiency
4. Caching implementation
5. Technical best practices
"""
)
- UX Agent
ux_agent = make_judge(
persona="UX Designer Uma, an experienced user experience designer",
example_feedback="The navigation structure is confusing, and the mobile responsiveness needs work. Important CTAs are not prominently placed.",
criteria="""
1. Navigation usability
2. Mobile responsiveness
3. Visual hierarchy
4. User flow
5. Accessibility
"""
)
- Helper Agent
helper_agent = make_judge(
persona="Helper Harry, a research assistant who provides context and coordination",
example_feedback="Based on industry standards, this website needs improvements in several areas. Let me help coordinate our evaluation efforts.",
criteria="""
1. Industry standard compliance
2. Best practices implementation
3. Competitive analysis
4. Trend alignment
5. Overall effectiveness
"""
)
All the agents are now defined and ready to go. Also think of Helper Harry as the project manager who makes sure everything comes together nicely, it’s there to make sure every agent works as they are supposed to!
Create a Workforce
A workforce is a collection of agents. Agents present in a workforce can communicate and interact with each others. To create a workforce we define it using ‘Workforce(“name of workforce”)' as shown below. Then we can simply add agents to it by using ‘.add_single_agent_worker’ command, this can be cascaded together to add multiple agents at once.
# Create workforce
workforce = Workforce('Website Evaluation Team')
# Add agents to workforce
workforce.add_single_agent_worker(
'SEO Expert Sarah (Judge), a meticulous SEO specialist who evaluates search engine optimization aspects',
worker=seo_agent,
).add_single_agent_worker(
'Content Creator Chris (Judge), a content strategist who evaluates content quality and structure',
worker=content_agent,
).add_single_agent_worker(
'Performance Pete (Judge), a technical expert who evaluates website performance and optimization',
worker=performance_agent,
).add_single_agent_worker(
'UX Designer Uma (Judge), a UX specialist who evaluates user experience and interface design',
worker=ux_agent,
).add_single_agent_worker(
'Helper Harry (Coordinator), a research assistant who coordinates evaluation efforts and provides context',
worker=helper_agent,
)
Now all the agents are added to the workforce, now they communicate with each other and give an output, the only thing remaining is assigning a task to this workforce.
Assigning a task to this Workforce
Finally, we create a task and pass it to the workforce
# Create evaluation task
task = Task(
content="""Evaluate this website comprehensively. Process:
1. Each judge should analyze the website based on their expertise
2. Give specific scores and recommendations
3. Helper should coordinate and summarize findings
4. Provide a final evaluation with actionable improvements""",
additional_info=str(scraped_content),
id="website_evaluation"
)
Here, the scraped content is the content that we fetched from the website that we wanted to evaluate. It was done by using a web-scraper.
Executing the task
result = workforce.process_task(task)
print("\n=== Website Evaluation Results ===")
print(result.content)
Finally after making the judges, adding different personas to them, adding them to a workforce and assigning them the task, we run the agent-society and get the results.
Worker node 136631569357648 (SEO Expert Sarah (Judge), a meticulous SEO specialist who evaluates search engine optimization aspects) get task website_evaluation.0: SEO Expert Sarah: Analyze the website's SEO aspects, including keyword optimization, meta tags, and overall search engine visibility. Provide specific scores and recommendations.
======
Reply from Worker node 136631569357648 (SEO Expert Sarah (Judge), a meticulous SEO specialist who evaluates search engine optimization aspects):
**SEO Analysis of Fingow (https://fingow.in)**
**1. Meta Tags Optimization: 1/4**
The only meta tag present is the viewport tag, which is essential for responsiveness but does not contribute to SEO. There is no meta description or title tag provided. A well-crafted meta title and description are crucial for search visibility and click-through rates.
**Recommendations:**
- Create a unique and engaging meta title that includes primary keywords.
- Write a compelling meta description that summarizes the page content and includes relevant keywords to improve click-through rates.
---
**2. Keyword Usage and Placement: 2/4**
The headings include various relevant phrases such as "Get a Loan from Anywhere" and "Elevate Your Finances". However, there is a lack of targeted keyword placement within the text content, which appears to be absent.
**Recommendations:**
- Conduct keyword research to identify high-volume keywords related to the services.
- Include these keywords naturally in the content and stage them throughout the headings and subheadings for better search visibility.
---
**3. URL Structure: 3/4**
Assuming the URL is straightforward and reflects the brand name, it is simple and user-friendly. A good URL structure, if continued with descriptive paths for the various services offered (e.g., /car-loan, /home-loan), would improve clarity and SEO benefits.
**Recommendations:**
- Maintain a clean and descriptive URL structure for all pages on the site. This includes utilizing keywords related to the content for better search relevance.
---
**4. Internal Linking: 2/4**
With two links present on the site, internal linking could be improved significantly. Internal links help boost SEO by distributing page authority and enabling better crawling by search engines.
**Recommendations:**
- Add more internal links to connect related content on the website. This could enhance user engagement and help with SEO performance.
The full output can be seen here: https://qb9.in/Blogs/camel
Conclusion
And there you have it! We built different teams of specialized agents to handle a range of tasks, from planning weekend trips to reviewing websites. Each agent worked together with a shared goal, making it easier to handle complex jobs efficiently.
In our use case at KubeNine, CAMEL-AI not only saved time and manual effort but also provided structured evaluations. By creating specialized agents, each with unique expertise we were able to replicate a real-life evaluation panel. The integration of these agents made it easy to simulate intelligent teamwork, something that would’ve taken a lot of time if done manually.